RADARs
Responsible AI Disclosure & Reporting System.
Project Brief
A web application streamlines AI transparency reporting for early-stage AI startups by combining smart templates with real-time guidance. This enables startups to focus on their priorities while staying informed with knowledge of potential risks.
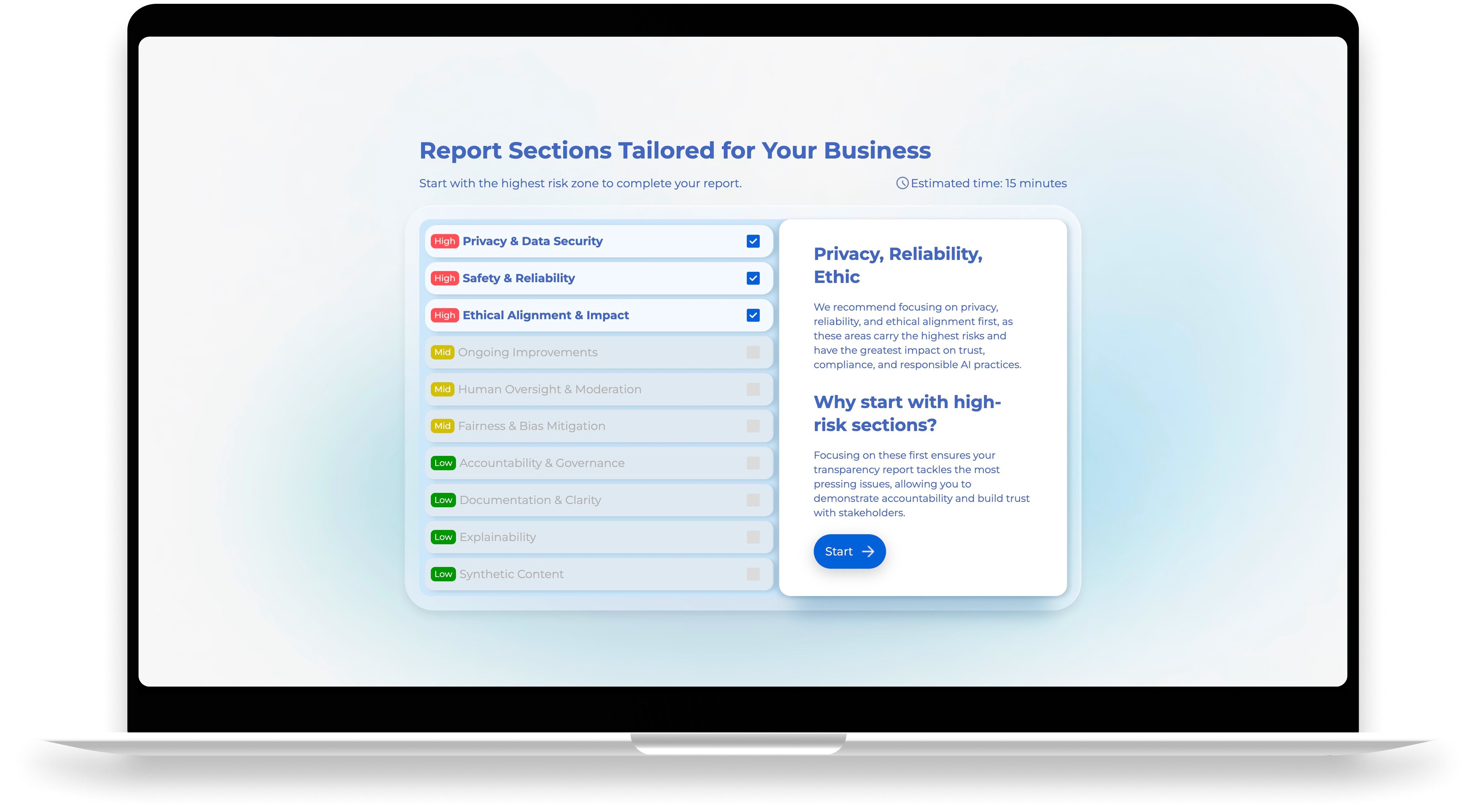
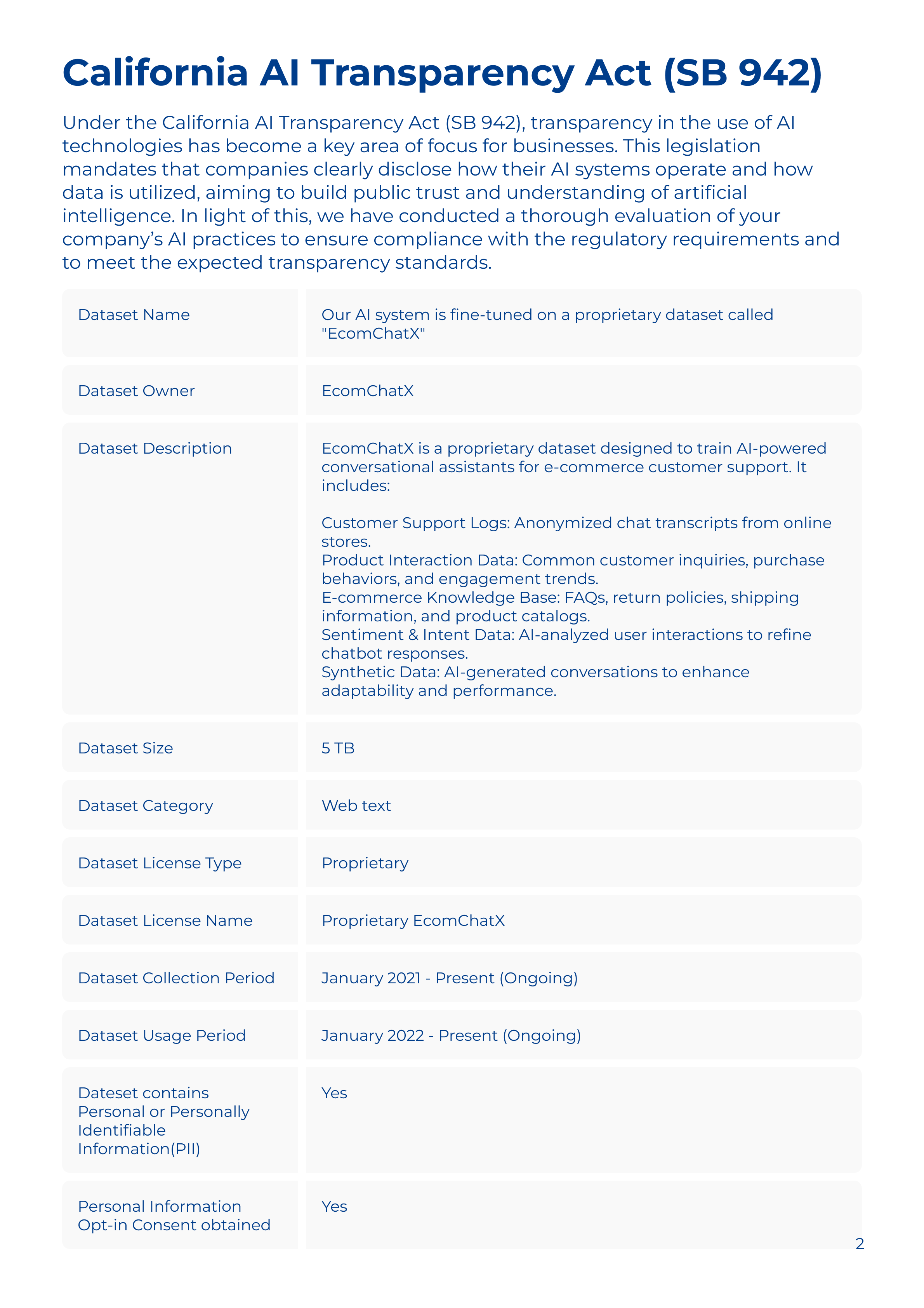
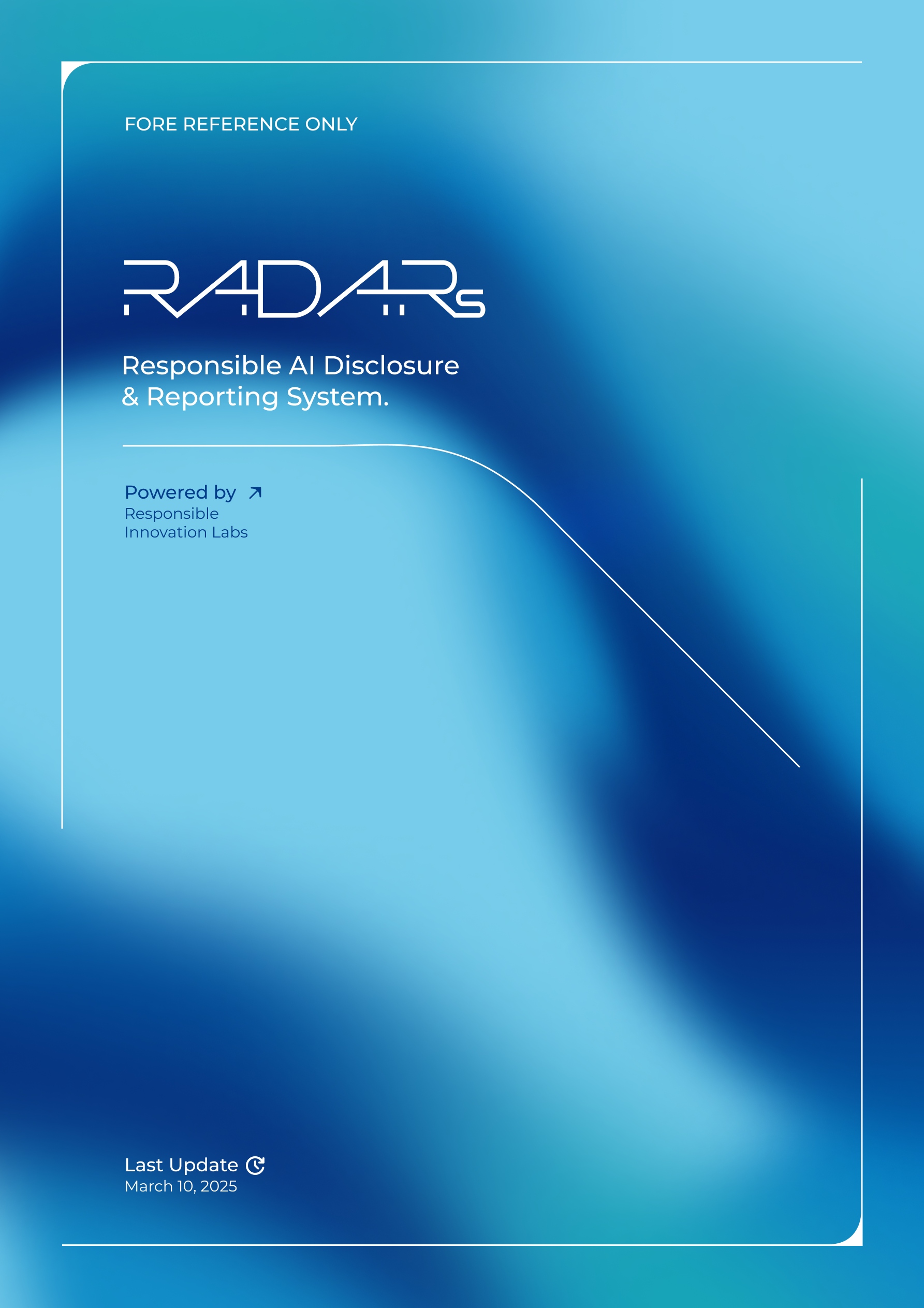
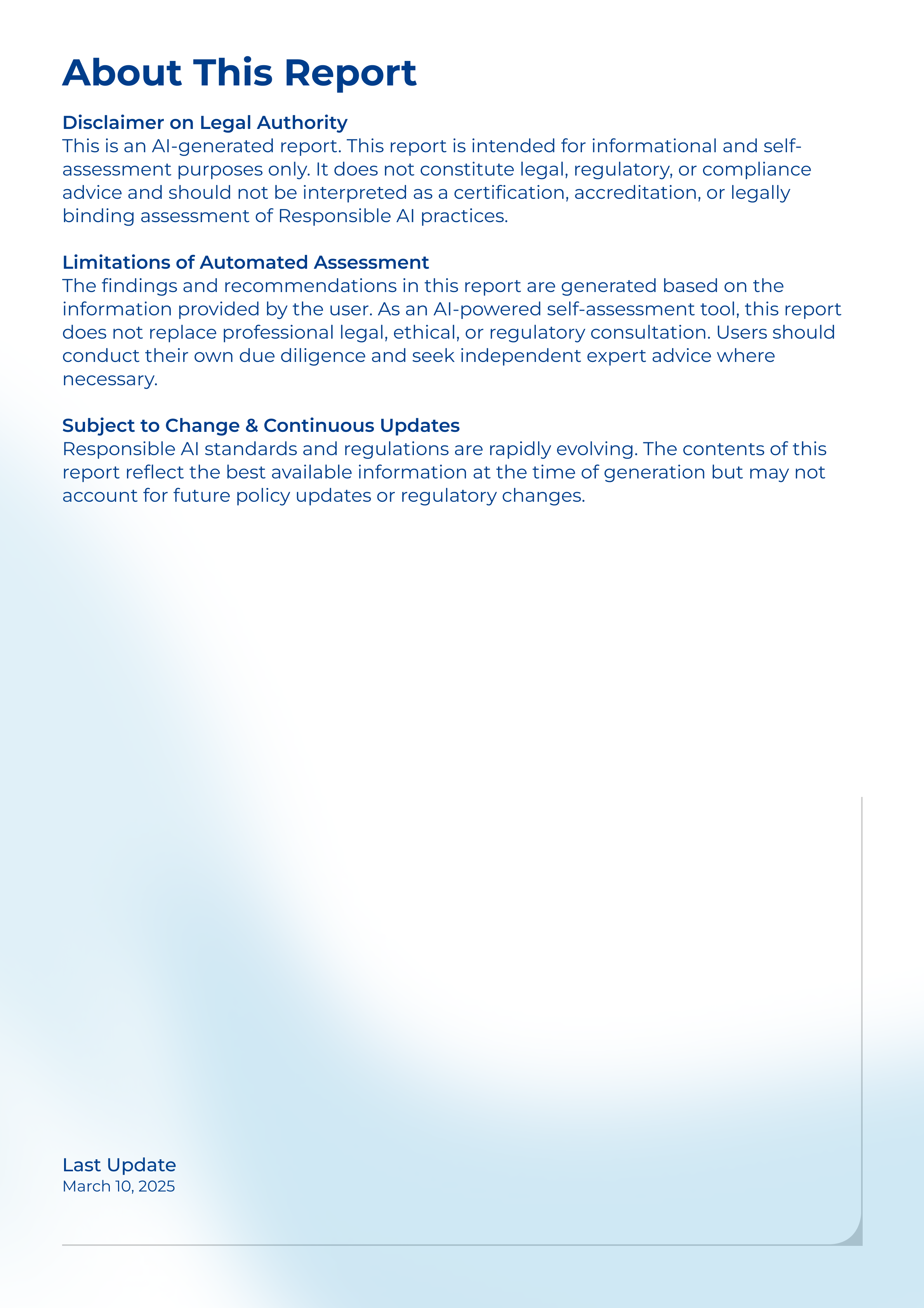
Sponsored By

My Role
UX Researcher & Designer
Front & Back-end Developer
Front & Back-end Developer
Project Type
To B Team Project
Time Frame
Sep 2024 - Mar 2025
Tools Used
Cursor, Webflow, Zapier, Figma, Adobe Photoshop, Lottie
Problem Statement
In an increasingly competitive startup landscape, survival is the top priority for AI startups, leaving little time and resources for ensuring transparency in AI operations. However, the lack of transparency increases business risks, making it harder to attract investors and build long-term trust.
40%
of startups reduced scale after AI transparency incidents
18+
months are usually required to rebuild trust with clients
Moreover, existing frameworks and toolkits for responsible AI practices are difficult to use and quickly become outdated, leaving startups without practical, up-to-date solutions.As a result, many AI startups prioritize survival over compliance, overlooking risks until they escalate into costly business threats.
Solution & Impact
Our tool empowers early-stage AI startups to not just survive but thrive. With insights into potential risks, our tool helps them make informed decisions that enhance investor appeal and long-term success. Once perceived as costly and time-consuming, transparency is now accessible and actionable, making it an asset rather than a burden.
Our web application streamlines AI transparency reporting for early-stage startups by combining smart templates with real-time guidance. This enables startups to focus on their priorities while staying informed with knowledge of potential risks.
Step 1: Basic Input
Users enter information about their industry, AI base model, use case(s), and audience.
Step 2: Tailored Questions
Answer a set of customized questions about their AI practices like data handling, testing, etc.
Step 3: Generate Report
Generates a comprehensive transparency report that identifies risks and provides suggestions.
56x
Faster responsible AI learning & reporting process for AI startups
20k+
Legal consultation cost expexted to be saved for AI startups
2
Companies are helping test our solution
Process & Approach
September 2024
Research
Research
● Secondary research
● Field study and research
● 13 subject matter experts interviews
● Field study and research
● 13 subject matter experts interviews

Insights:
● "Responsible AI is important!"
● There're already countless Responsible AI Frameworks on the market
● "Responsible AI is important!"
● There're already countless Responsible AI Frameworks on the market
November 2024
Pivot & Define
Pivot & Define
● Research questions
● Product direction
● Problem scope
● Product direction
● Problem scope
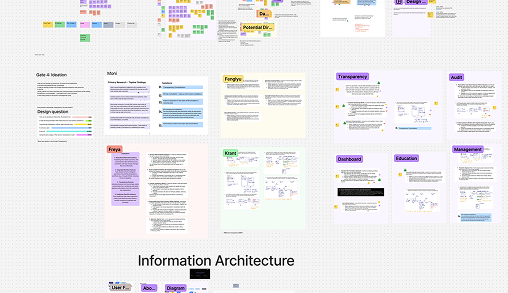
Insights:
● AI startups do not care about Responsible AI. They put development and survival the top priority.
● Transparency on AI practice (part of Responsible AI) is already a trend from legal perspective.
● Helping startups improve transparency on AI practice could be our breaking point.
● AI startups do not care about Responsible AI. They put development and survival the top priority.
● Transparency on AI practice (part of Responsible AI) is already a trend from legal perspective.
● Helping startups improve transparency on AI practice could be our breaking point.
The “Catch-22” for AI Startups:
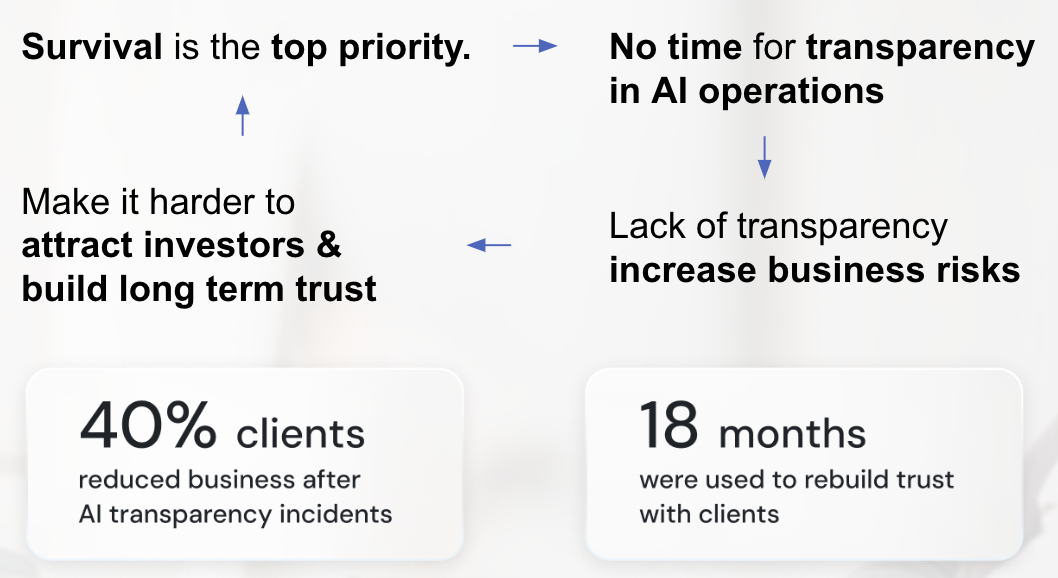
Why existing tools don't work:
● No mandatory laws yet for AI companies in the U.S.
● Frameworks from large corporations act more as legal shields, often spanning 50-200 unreadable pages.
● No mandatory laws yet for AI companies in the U.S.
● Frameworks from large corporations act more as legal shields, often spanning 50-200 unreadable pages.
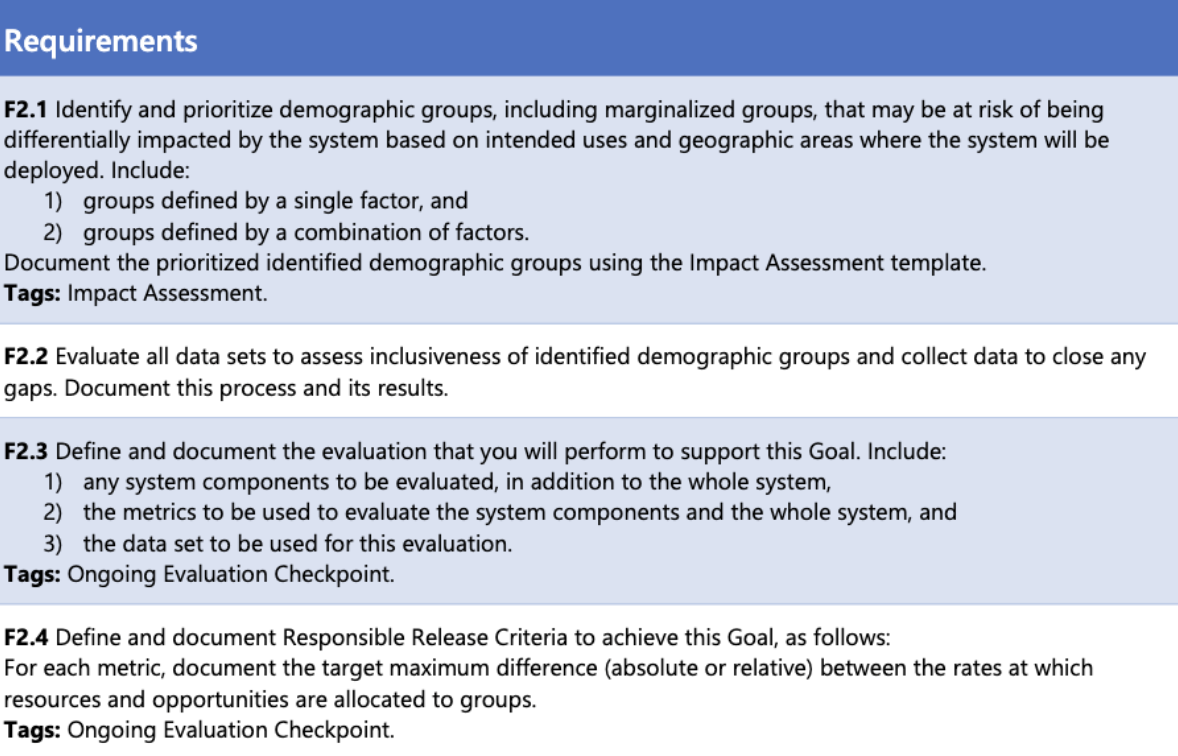
December 2024
Prototype
Prototype
● System architecture
● User flow
● Low to high fidelity UX/UI
● Frontend & backend implementation
● User flow
● Low to high fidelity UX/UI
● Frontend & backend implementation
Feburary 2025
Iterate
Iterate
● 4 prototype usability tests
● 3 design iteration sprints
● 5 functional usability tests
● 6 Stress tests
● Debug sprint sessions
● 3 design iteration sprints
● 5 functional usability tests
● 6 Stress tests
● Debug sprint sessions
March 2025
Deliver
Deliver
● Documentation
● Cloud environment setup
● Asset Handover (design Assets, codebase, technical documentation)
● Open Pitch & Poster Session
● Performance monitoring & feedback collecting
● Cloud environment setup
● Asset Handover (design Assets, codebase, technical documentation)
● Open Pitch & Poster Session
● Performance monitoring & feedback collecting
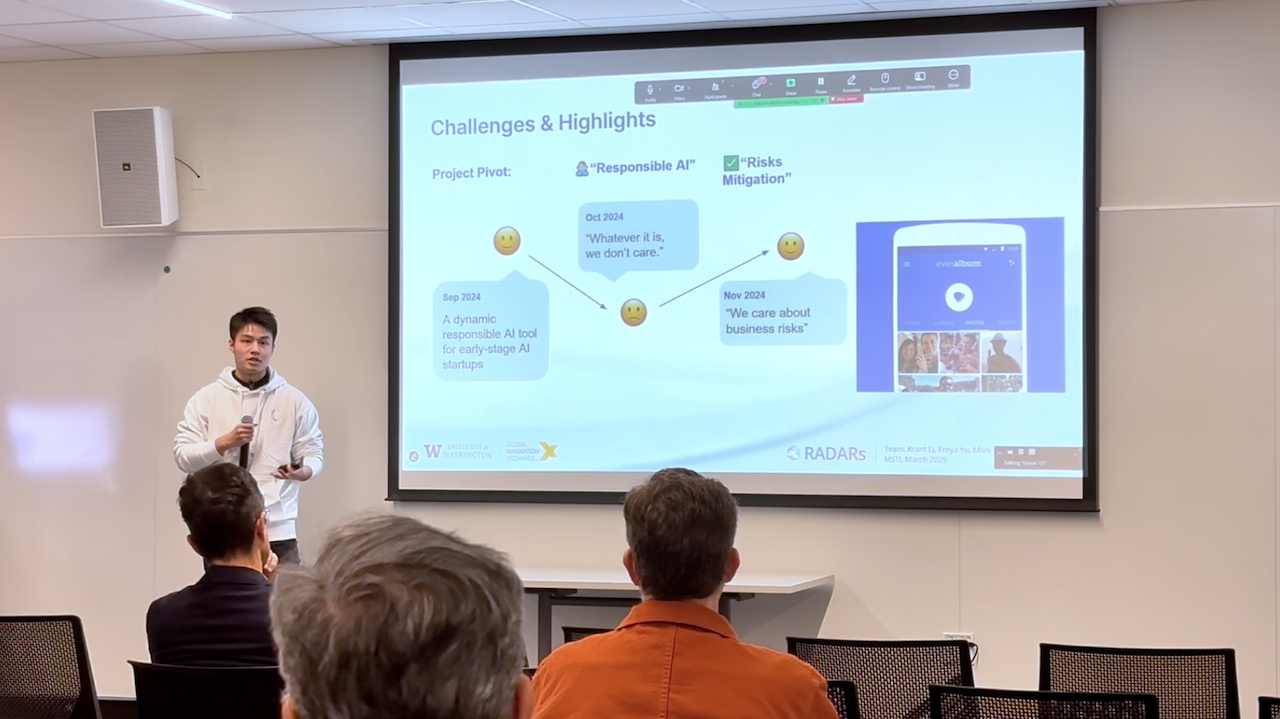